Cohort Analysis: Use-cases
Now that we have a basic understanding of how one can analyze patterns in a cohort table, here are a few compelling scenarios which make cohort analysis an essential tool for all marketers:
1. Validate Your Hypotheses About User Behavior
Any user behavior can be attributed to several factors. However, for a marketer to conclude that a specific element is responsible for the observed behavior, each hypothesis needs to be tested over some time.
Use-case
Let’s take the example of a mobile e-commerce app to understand this better. For instance, you want to analyze how long it takes a user to purchase after viewing a product, without being influenced by any follow-up communication from you.
Here’s how cohort analysis can help
You could do a simple cohort analysis of users who viewed a product and purchased it, over a period of 10 days. The table would look something like this:
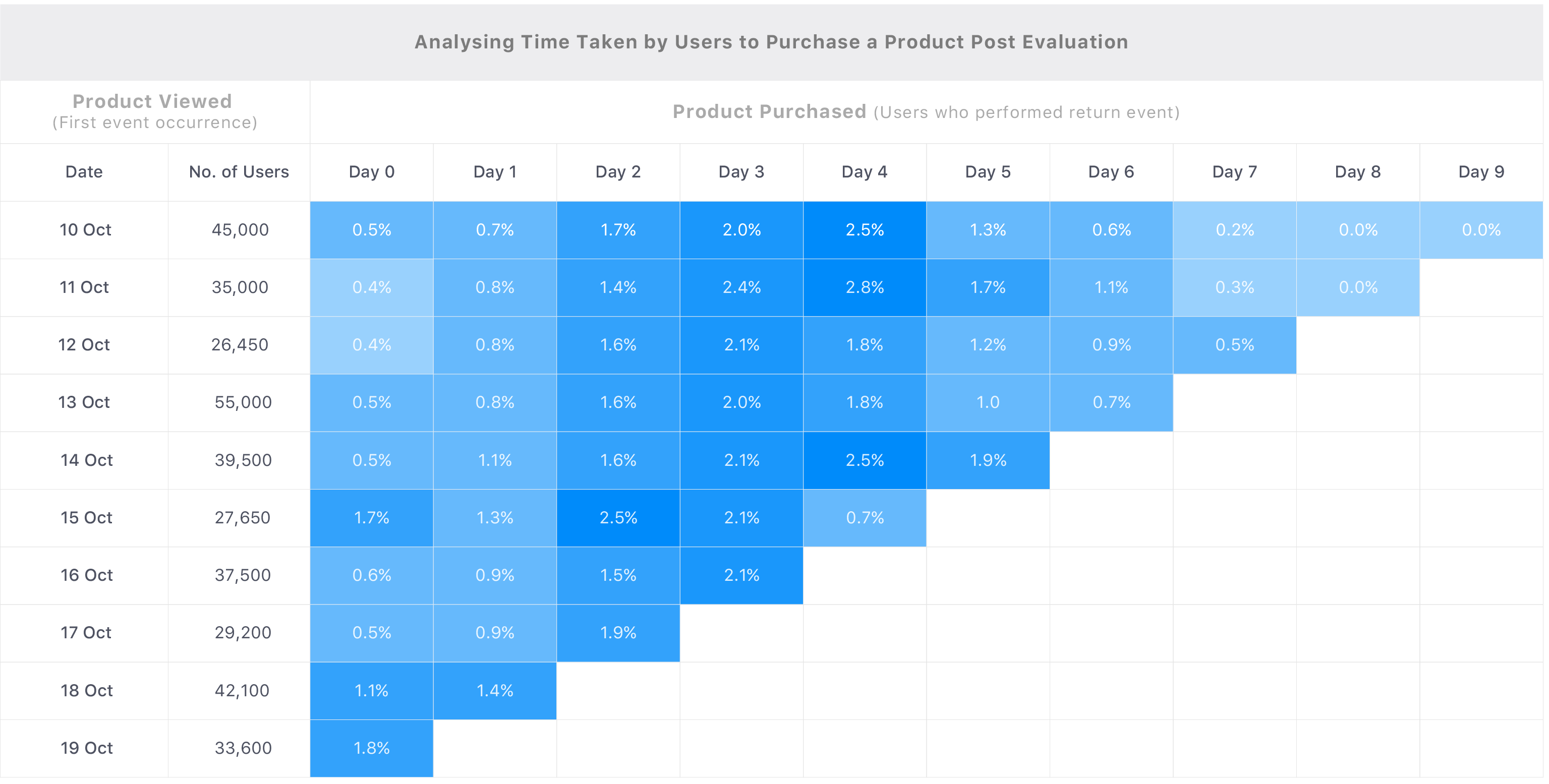
Click to enlarge
Results
A quick analysis of the broad trends of the pockets of deep blue in the table reveals that across all cohorts, users seem to take 2 - 4 days to purchase a shortlisted product.
Here are a few hypotheses we came up with to understand this peculiar behavior:
Hypothesis 1: The users are not sure that they are getting the best price on your site and are thus, evaluating other options.
Hypothesis 2: The products are of high value and are generally purchased once in a long period of time. Hence, the users need some time to decide if they want to go ahead with the purchase.
Hypothesis 1 is a mix of a product problem and the trust your brand is yet to build amongst its users.
While Hypothesis 2 is a simple decision-making problem at the user’s end. An easy way to test Hypothesis 2 is by implementing a conversion campaign strategy to help the users decide faster. You can send them persuasive messages, pushing them to make the purchase.
Let's dig deeper into hypothesis 2
You could devise a multi-channel strategy and reach out to the user after an hour of exiting your app through a push notification, reminding them that the awesome product they viewed is waiting for them!
Alternatively, if someone has disabled push notifications, you could send them an email or an SMS. The second nudge is sent out the next day and a third one, the day after.
Let’s run our cohort analysis again.
Let’s say that you implement this strategy on a test basis for 7 days. On day 8 you do a cohort analysis to test your hypothesis and this is what you see:
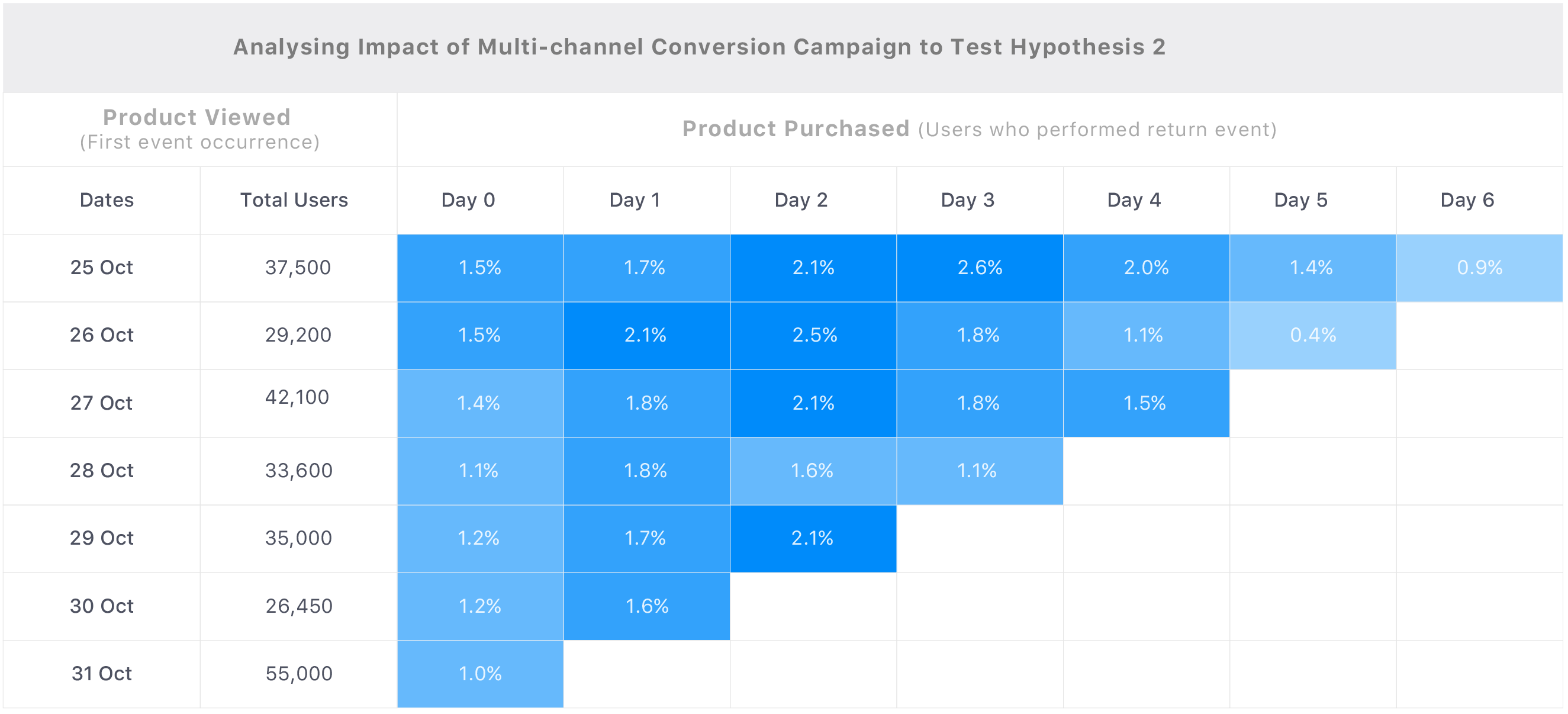
Click to enlarge
On analyzing the above cohort table you will find that there is a considerable improvement in the purchase rates for Day 0 - Day 2, proving our hypothesis right!
2. Identify Channels Through Which Premium Customers Have Been Acquired
Use-case
Let’s say that you run a gaming app which has a high uninstall rate. As a marketer, you have no problem in acquiring new users - but around 70% of them abandon or uninstall your app within a month. This adds to your cost of acquisition and marketing efforts.
But on the brighter side, 30% of your users are still around! Hence, by identifying your loyal users and understanding where they came from, you can focus your acquisition marketing efforts on those channels.
Here’s how cohort analysis can help
You can create a cohort of users who regularly play a game, split that data by the channel they were acquired through, and view the data over a period of 8 weeks.
Your cohort would look something like this:
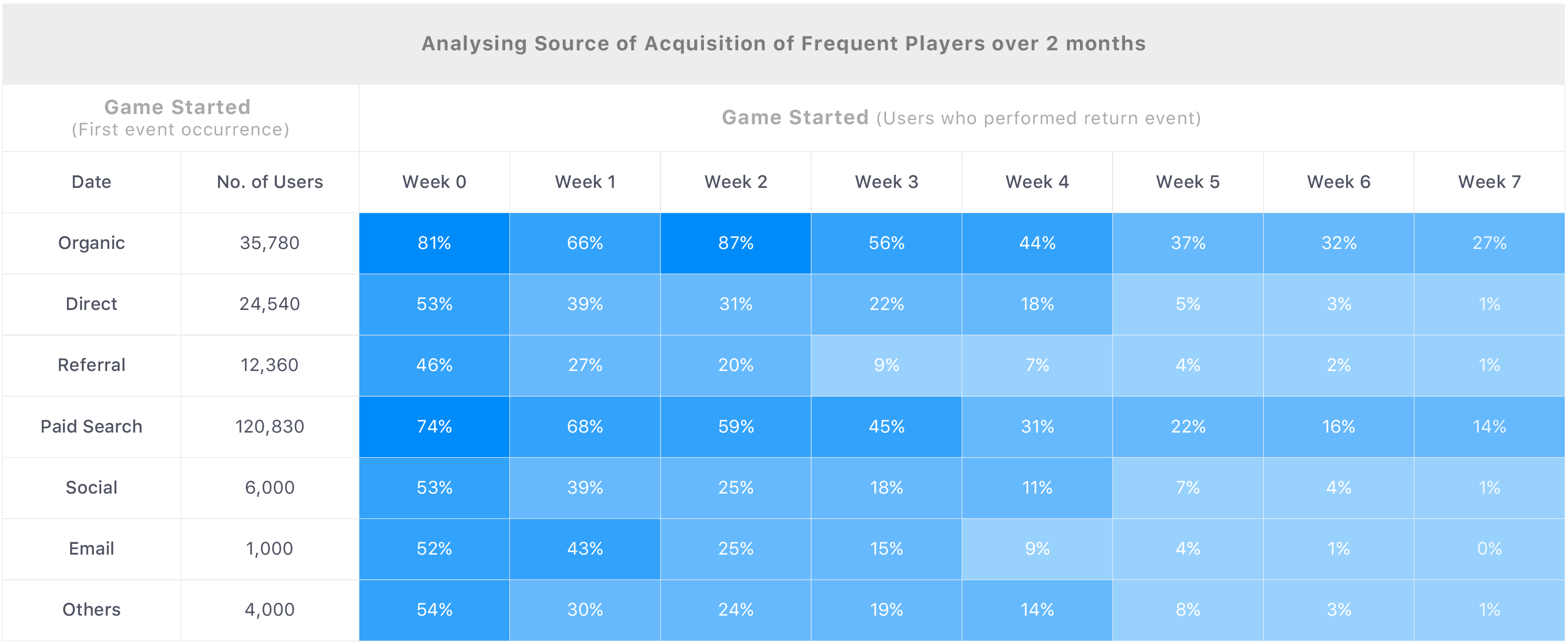
Click to enlarge
Results
A quick glance over this cohort table will tell you that users who found your gaming app through Organic Search and Paid Search stayed around the most.
- This means that you should run more paid search campaigns, for the same keywords you targeted previously, and invest in improving your gaming app’s SEO to acquire high intent users.
Similarly, users who found your gaming app through sources like Referral and Email exhibit a considerable drop in engagement post Week 2.
- This implies that these channels are not contributing high intent users to your app you will need to work on reaching out to the right target audience through these channels.
3. Separate Growth Trends from User Engagement Trends
Another important problem solved by cohort analysis is that it allows marketers to get a clear picture of user engagement. When looking at plain numbers, the lack of activity of a group of users can easily be masked by a growing user base.
Use-case
Let’s say that you run an e-commerce website and occasionally run acquisition campaigns to bring in more users. Campaign A was run 15 days ago and brought in 10,000 users to your site. Campaigns B was run 7 days ago and brought in 20,000 users.
Now, when you look at the Events section of your dashboard, you see that the event, Checkout- Completed has occurred a higher number of times than your monthly average, over the last 15 days. This indicates a steady growth - but is this reason enough to rejoice?
Let’s dig deeper.
Here’s how cohort analysis can help
On doing a cohort analysis of users against repeat purchases over the last 15 days, split by acquisition source, this is what you see:
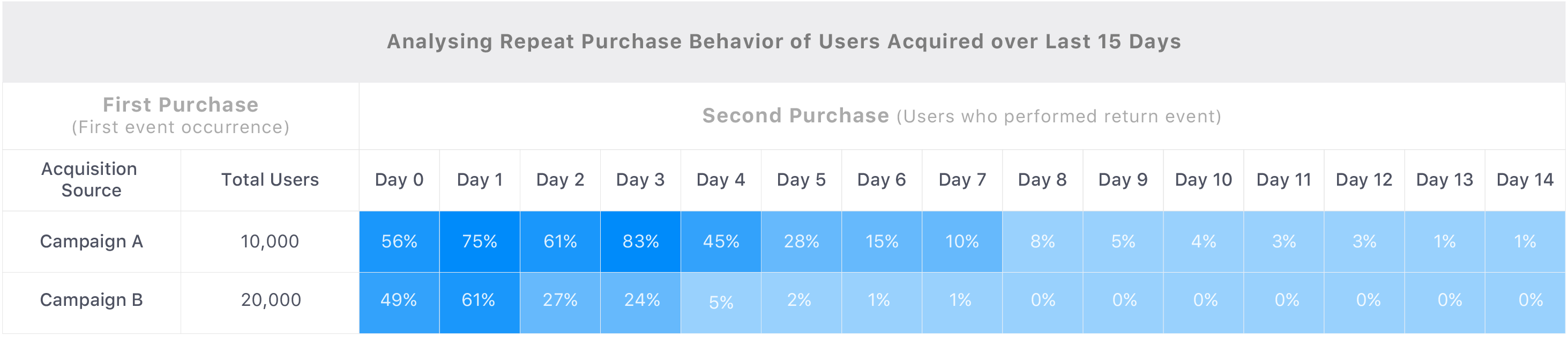
Click to enlarge
Results
Even though Campaign B helped you acquire double the number of users, the quality of users acquired through Campaign A is better as they seem to be engaged for a longer duration.
If it were not for cohort analysis, you would have never figured this out and would have probably continued to run more acquisition campaigns as Campaign B. This leads to a few questions:
- Why did the users, acquired through Campaign B, not stay around for longer?
- Were most of these users deal shoppers?
- Are these users different from the users acquired through Campaign A, regarding their needs and expectations from your website?
- Did you send the users acquired through Campaign B, the same retention marketing campaigns as users acquired through Campaign A?
- How can you re-engage the lost users?
4. Understand the Needs of Your User Base
Understanding a constantly changing user base is one of the biggest challenges faced by marketers today. Volatility in the composition of any online business’ user base can be attributed to several factors such as seasonal shoppers/deal-shoppers, one-time shoppers lost to competitors, and so on.
This makes it difficult for marketers to discern different patterns of user behavior. While most analytics platforms will give you a broad demographic breakup of your user base and their interests, they do not give much insight into their behavior.
This is where cohort analysis can help, let's understand this better with a practical use-case.
Use-case
Let’s say that you run an e-commerce site which frequently runs end-of-season sales. As a result, you experience high purchase rates during the sale period, but once the sale ends, business is back to usual.
While this is typical user behavior, we are missing out on a very important aspect - the after effect of the sale on your business. Usually, an end-of-season sale has the following features:
They are supported by extensive marketing campaigns, aimed at creating awareness and acquiring new users.
They are supported by re-engagement campaigns, aimed at bringing lost users back to grab products they were interested in, at a discounted rate.
They are supported by cross-selling campaigns, aimed at engaging high intent users.
In each case mentioned above, if a customer has a good experience with your end-of-season sale, then it’s highly likely that they will return on a later date to shop for more.
But do they?
Let’s do a cohort analysis to find out.
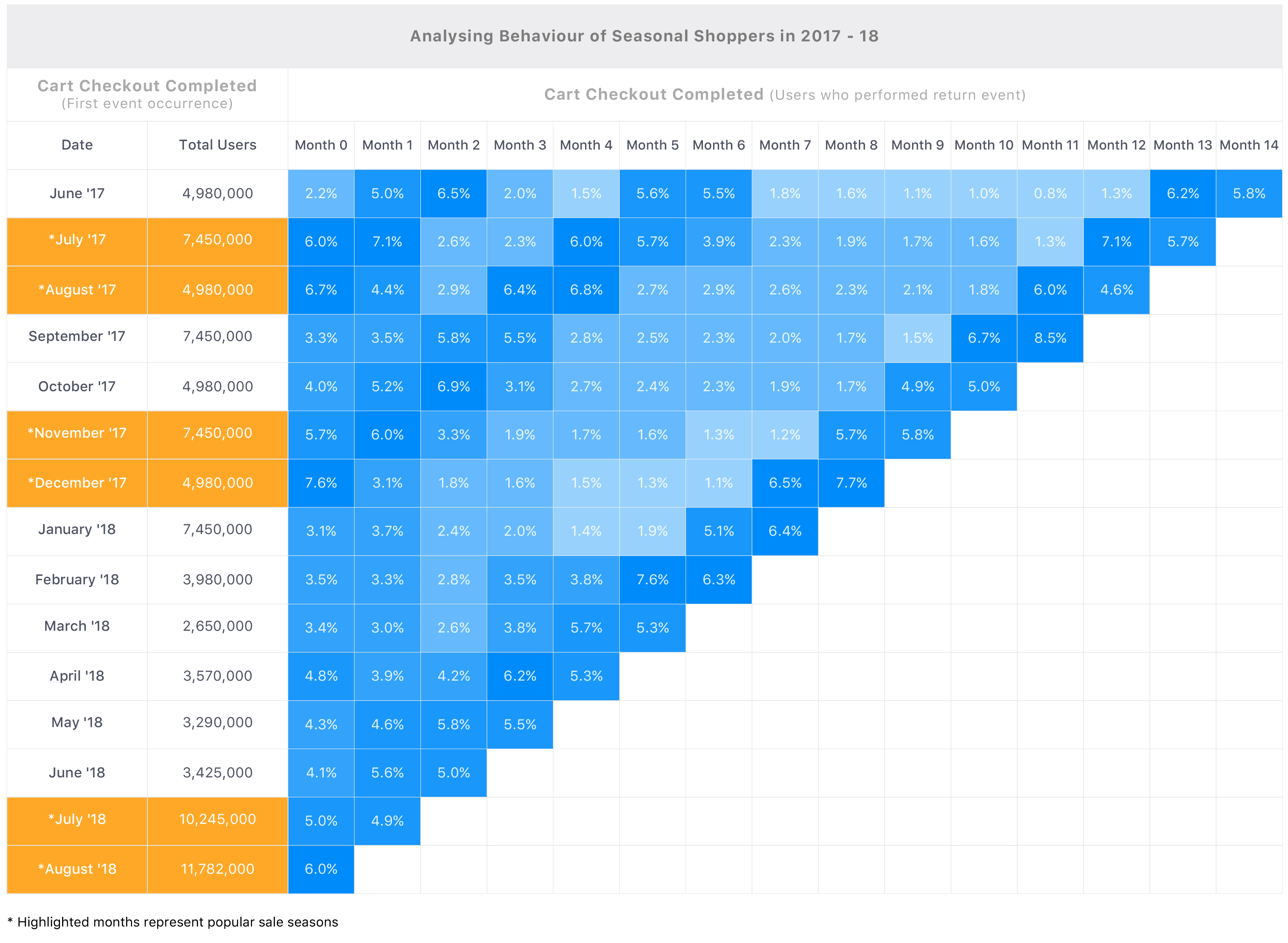
Click to enlarge
Let’s assume that you run two seasonal sales every year; The first one is an End-of-Summer Sale from July to August and the other one is a Winter-Christmas Sale from November to December. On doing a cohort analysis of repeat purchasers over a period of 15 months, you find that your seasonal sales have been doing pretty well in promoting repeat purchases.
Results
Going by the pockets of deep blue in the table above, one can decipher the following user behavior:
- Irrespective of which month your users made their first purchase in, as high as 8.5% of them. return to your site to grab seasonal deals. This seems to be largely true for all your seasonal sales.
- As high as 7.7% of users who made their first purchase in a sale, make return purchases in the consecutive sales.
- As high as 3.9% of users who made their first purchase in a sale, made a repeat purchase in the non-sale months too.
- There is a considerably high difference between the repeat purchase rates of sale months and the non-sale months.
This means that your seasonal sales have played an important role in re-engaging customers and driving repeat purchases over a long period of time. Thus, you should consider experimenting with a Spring Season Sale in March to see if the trend of high repeat purchases continues!
5. Identify Churn Trends
A high churn rate is a big setback for all online business as it adds on to the cost of user acquisition. Generally, most marketers have to deal with that fact that churn is inevitable. But that doesn’t mean that it can’t be controlled. You can influence your users to stay around longer, and even become loyal customers by identifying factors which lead to churn and fixing them.
Use-case: Identifying churn patterns for a gaming app
Let’s say that you run a gaming app and are currently battling a higher than average uninstall rate.
Let’s do a quick cohort analysis to see how bad the situation is:
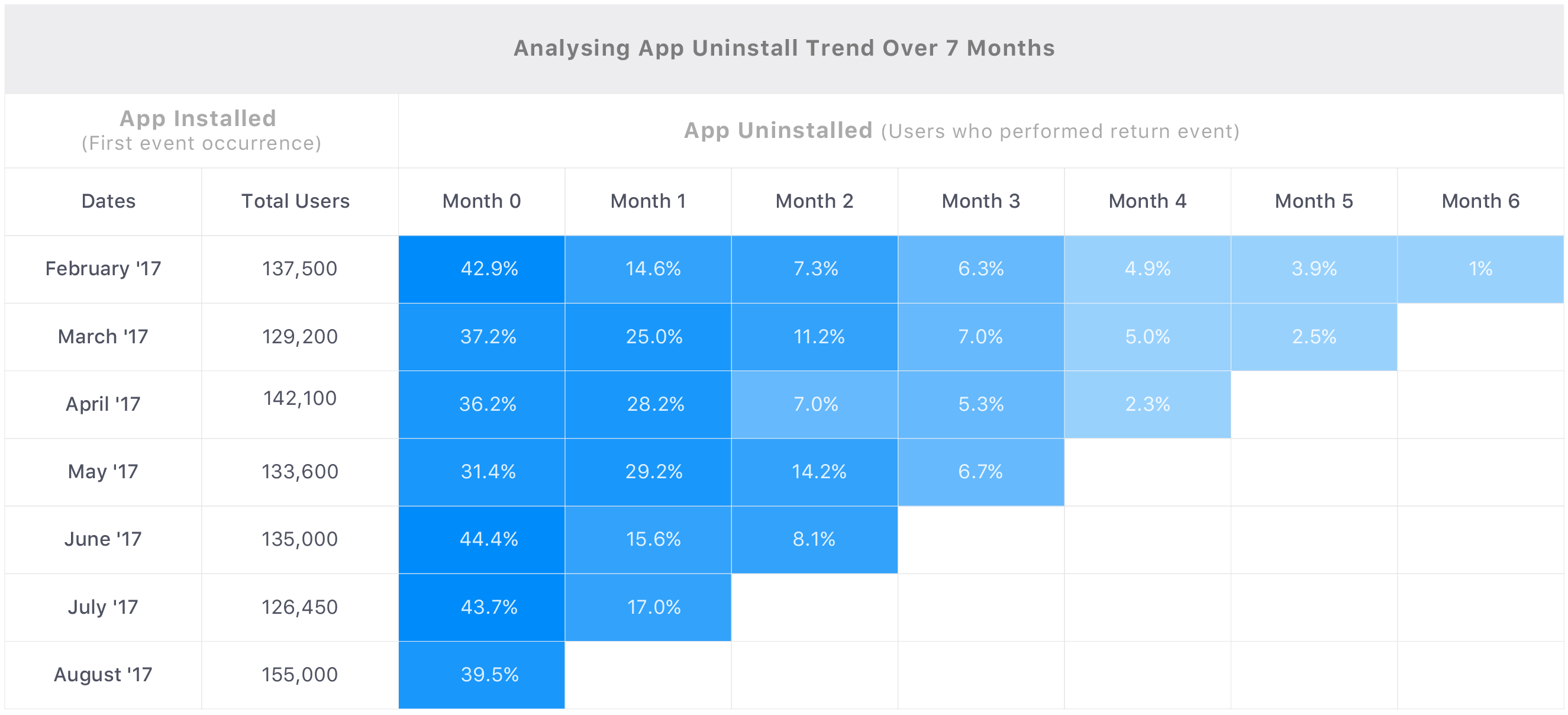
Click to enlarge
On analyzing users who have uninstalled in the last 7 months, you find that as high as 44% of them uninstalled the app within the first month itself! While the uninstall rate drops significantly in the second month for most users, it continues to be as high as 14% in the third month.
Another interesting thing revealed by the cohort table is that your gaming app is not sticky enough. On average, 68% of your acquired users churn within 4 months of installing the app. This could be due to several reasons. But had it not been for cohorts, it would have been extremely difficult to identify this pattern.
Reverse cohort analysis to dig deeper
In continuation of the use-case: Identifying churn patterns for a gaming app
Now let’s do a reverse cohort analysis (over a period of 2 months) to see how many of the users who uninstalled your gaming app, played a game before doing so.
This will help us dig deeper into what went wrong and how the user experience can be improved to keep the uninstall rates in check.
The table would look something like this:
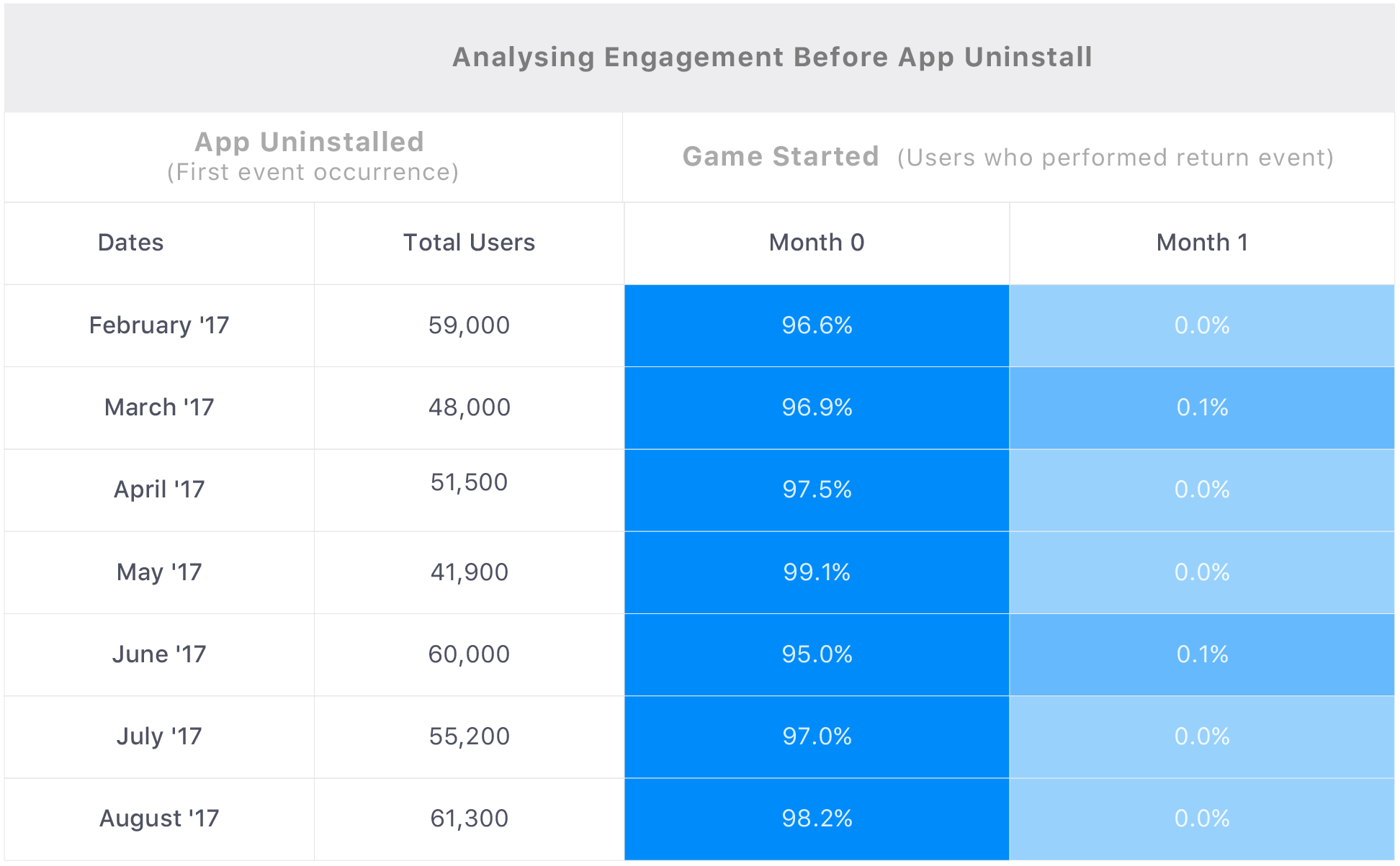
Click to enlarge
On analyzing the table above, It’s clear that upwards of 95% of the users played at least one game on your app before uninstalling it. This leads to a few hypotheses as to why the users uninstalled your app:
- The game was not at par with the user’s age group or interests.
- The game was not engaging enough.
- The app crashed while they were playing the game.
- The on-boarding experience was not the best.
And the list could go on.
As marketers, our job will be to list down all the relevant scenarios and test each one to reduce the uninstall rate.
But what about the remaining 5% who didn’t play a game?
A quick average of the engagement rates of all 7 months reveals that only 95.9% of the users actually played a game before uninstalling your app.
- Seems like the remaining users simply uninstalled the app without even exploring what it has to offer. Such statistics are generally worrying for retention marketers and product managers as it indicates that there is an underlying problem with the app’s communication or on-boarding process.
Another interesting thing revealed by the reverse cohort analysis is that 0.1% of users reinstalled your gaming app the next month and played a game.
- This is a win for you! Even though it’s a small number, it means that you can win back lost users by running retargeted campaigns on the right channels in the future.
If it weren’t for cohort analysis, would you have ever figured this out by yourself?
6. Gain Insights into Specific User Behavior
One of the most important value propositions of doing cohort analysis for marketers is that it allows them to ask very specific questions related to user behavior and analyze only the relevant data. This makes the insights actionable, allowing marketers like us to adapt to user needs and communicate more effectively.
So rather than looking at all your users as a single unit, you can break them down into cohorts to identify relevant behavioral patterns throughout their lifecycle. This can help you answer questions like:
- Which features do customers keep coming back to use?
- How does a particular cohort respond to certain retention marketing campaigns you send out to them? Does it lead to more conversions?
- How sticky is your product for new users (how soon users understand and adapt your product)?
Use-case
Let’s say that you want to understand how long it takes users to purchase a product, post evaluation. While doing a funnel analysis of the events which lead up to purchase will give you an insight into how many people don’t make a purchase - it will not help you understand the behavior of the people who made the purchase.
Here’s how cohort analysis can help
You can analyze users who first viewed a product and then purchased it, within an ideal period. This will help you get an idea of how long it takes a user to decide that they want a product.
Let’s analyze to understand behavior for 10 days.
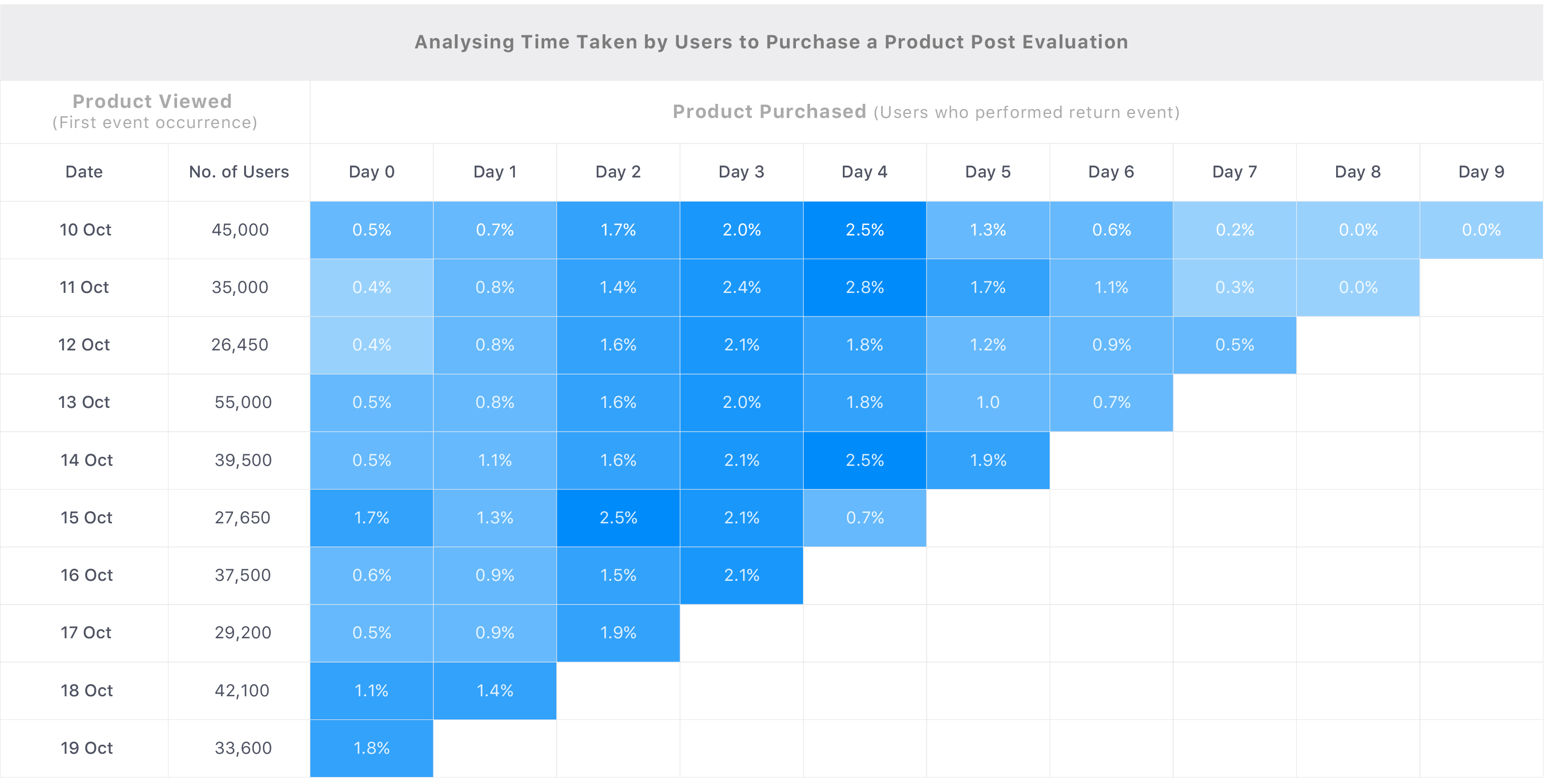
Click to enlarge
Results
Looking at the trend of pockets of dark blue in this cohort, one can decipher that most of the users make a purchase 2 - 4 days after evaluating a product. This interesting behavior could be attributed to several factors:
- You are sending these users reminders that the product they viewed is still up for grabs between day 2 and day 4.
- You are sending the users discount offers on the same product/related products on day 3 or day 4 (since these days show the highest purchases).
- The users are not sure that they are getting the best price on your site and are thus, evaluating other options (could be attributed to lack of trust, which is common in the case of new e-commerce sites).
- The products are of high value and are generally purchased once in a long period. Hence, the users need some time to decide if they want to go ahead with the purchase (typical in the case of sites selling high-end electronics and appliances).
Thus, depending on the kind of website/app, your hypothesis could vary.
The next step would be to establish why the users take 2 - 4 days to make the purchase and reduce the time gap, if possible.
7. Understand Conversion Patterns
While funnels do an excellent job of telling you how many users progressed from Event A to Event B - they don’t paint an elaborate picture. But cohorts do.
By analyzing users who moved from Event A to Event B, you can gain detailed insights into:
- How many hours, day or months did it take your users to perform this conversion?
- Did your marketing campaigns have an impact on the conversion rates?
- Were these users self-motivated to perform Event B, or did you have to motivate them with offers?
Let's understand this better with a use-case.
Use-case
Let’s say that you are promoting your content marketplace and are aggressively running campaigns to get new signups.
You run the first campaign from, June ‘17 to July ‘17 and acquire 80k visitors, of which 2.2% users signup.
You run a second campaign from September ‘17 to October ‘17 and acquire 100k visitors of which 2.5% users signup.
Here’s how cohort analysis can help
On December 1, 2017, you create a cohort of users who visited your site and signed up, over the last 6 months. This is what you see:
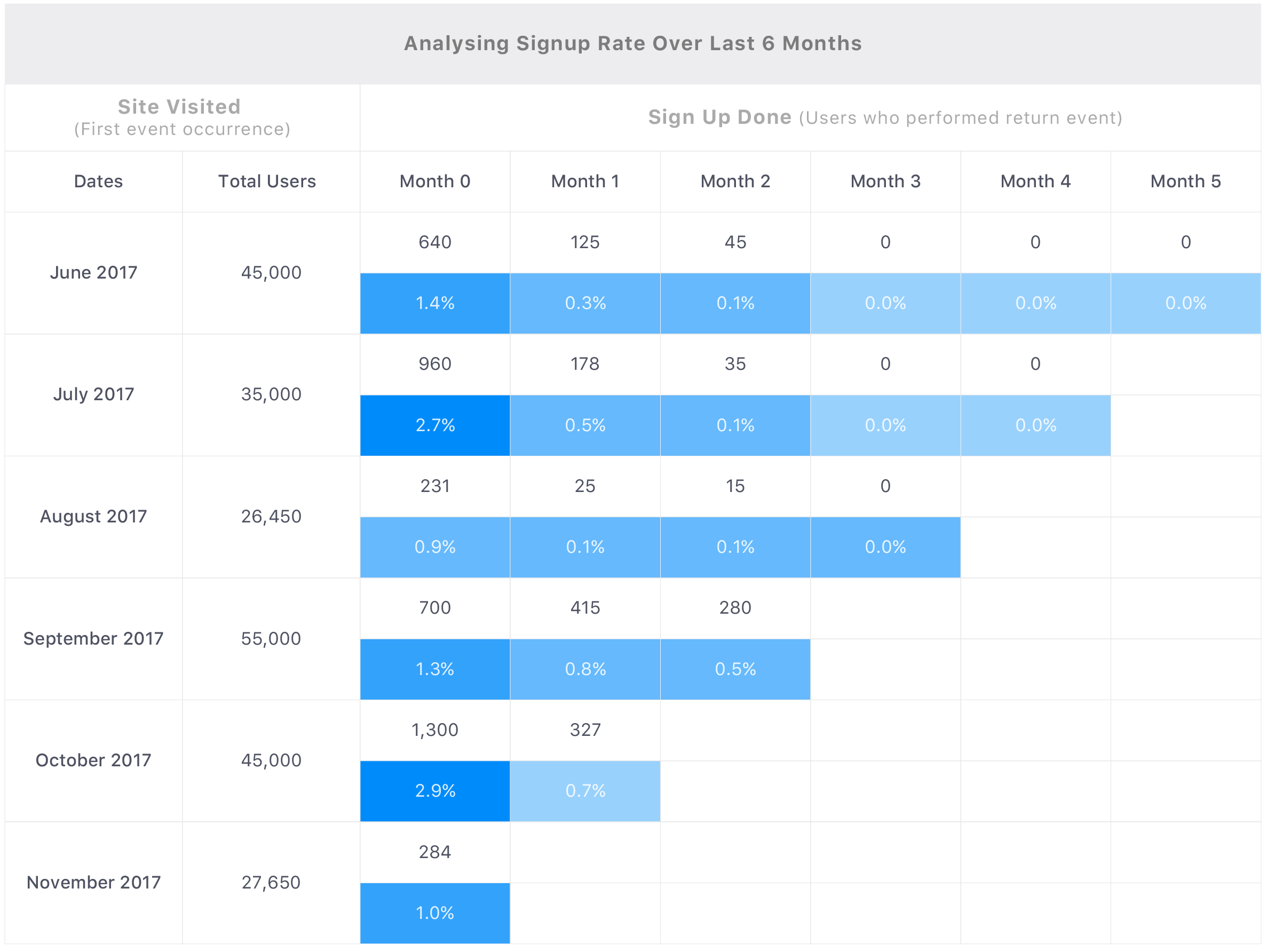
Click to enlarge
The analysis reveals that both your campaigns have had a larger impact than initially estimated. This is because some users signed up for your services in the following weeks/ months after visiting your website. As a result, they were not accounted for when we first calculated the signup rate.
But on calculating the signup rate immediately after running the marketing campaigns, this is what we see:
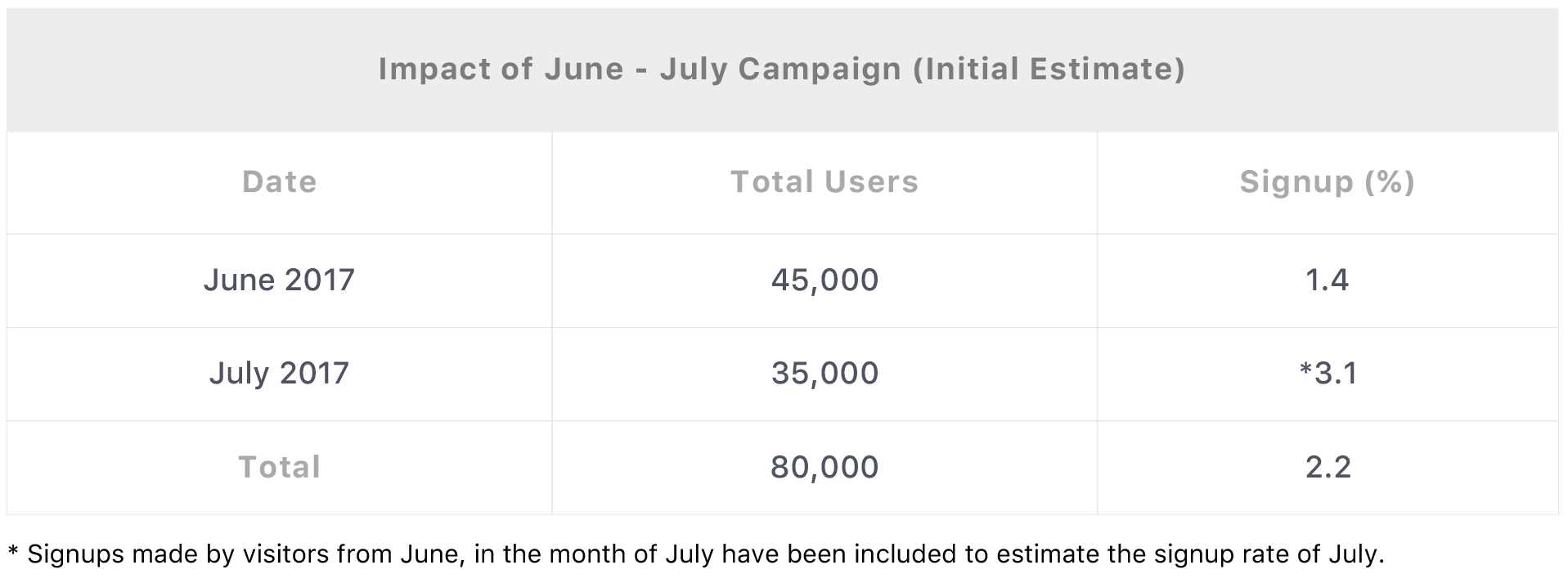
Click to enlarge
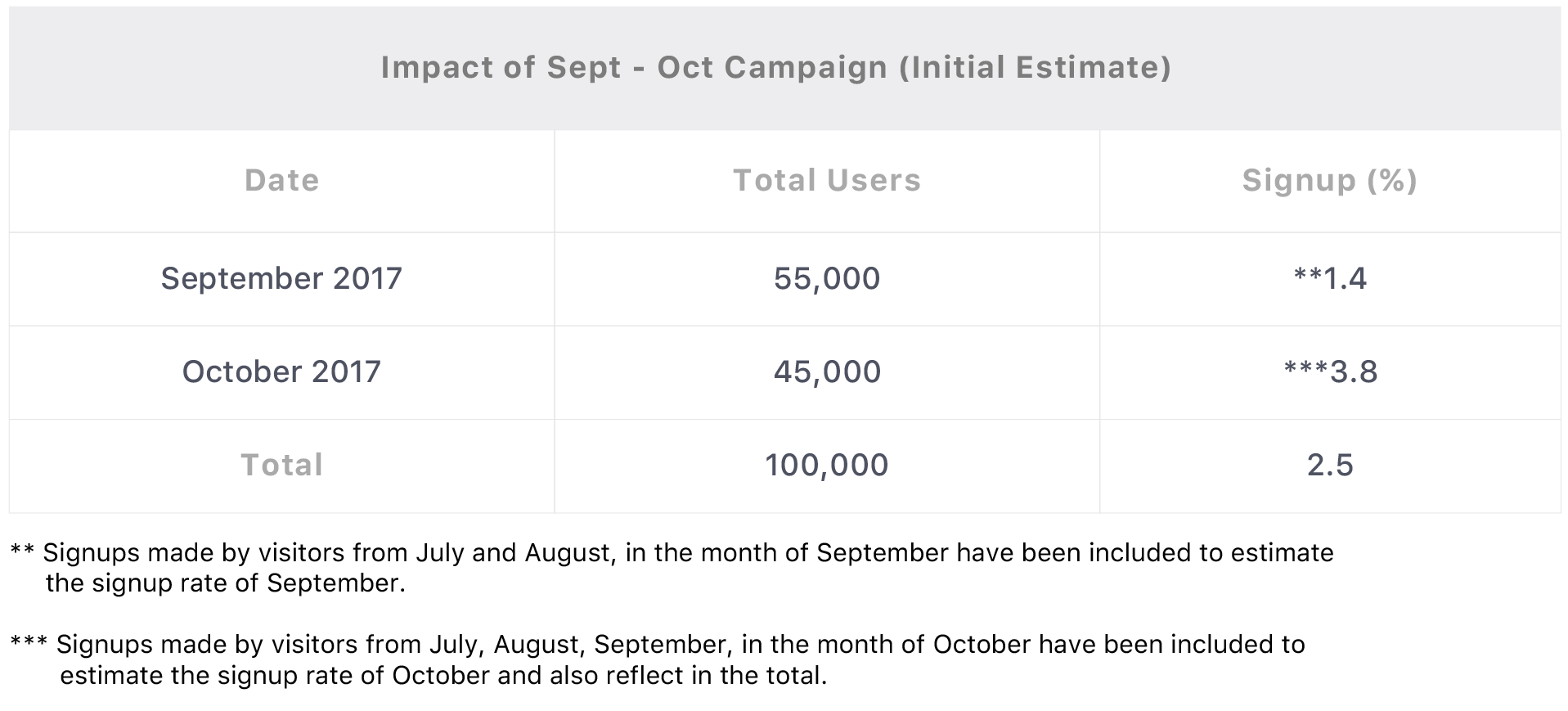
Click to enlarge
But the cohort analysis reveals a different picture, with higher signup rates than initially estimated.
Here are the revised signup rates for both the campaigns:
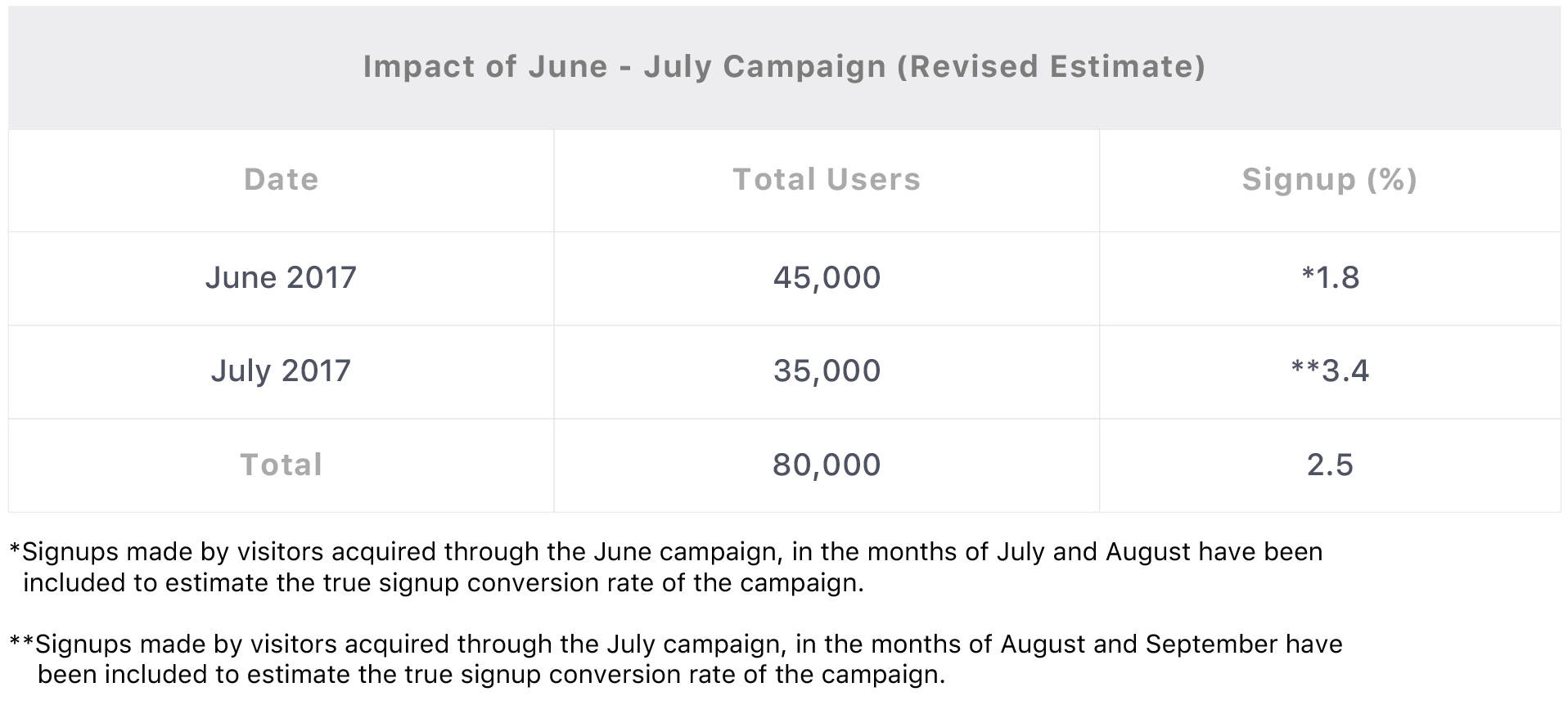
Click to enlarge
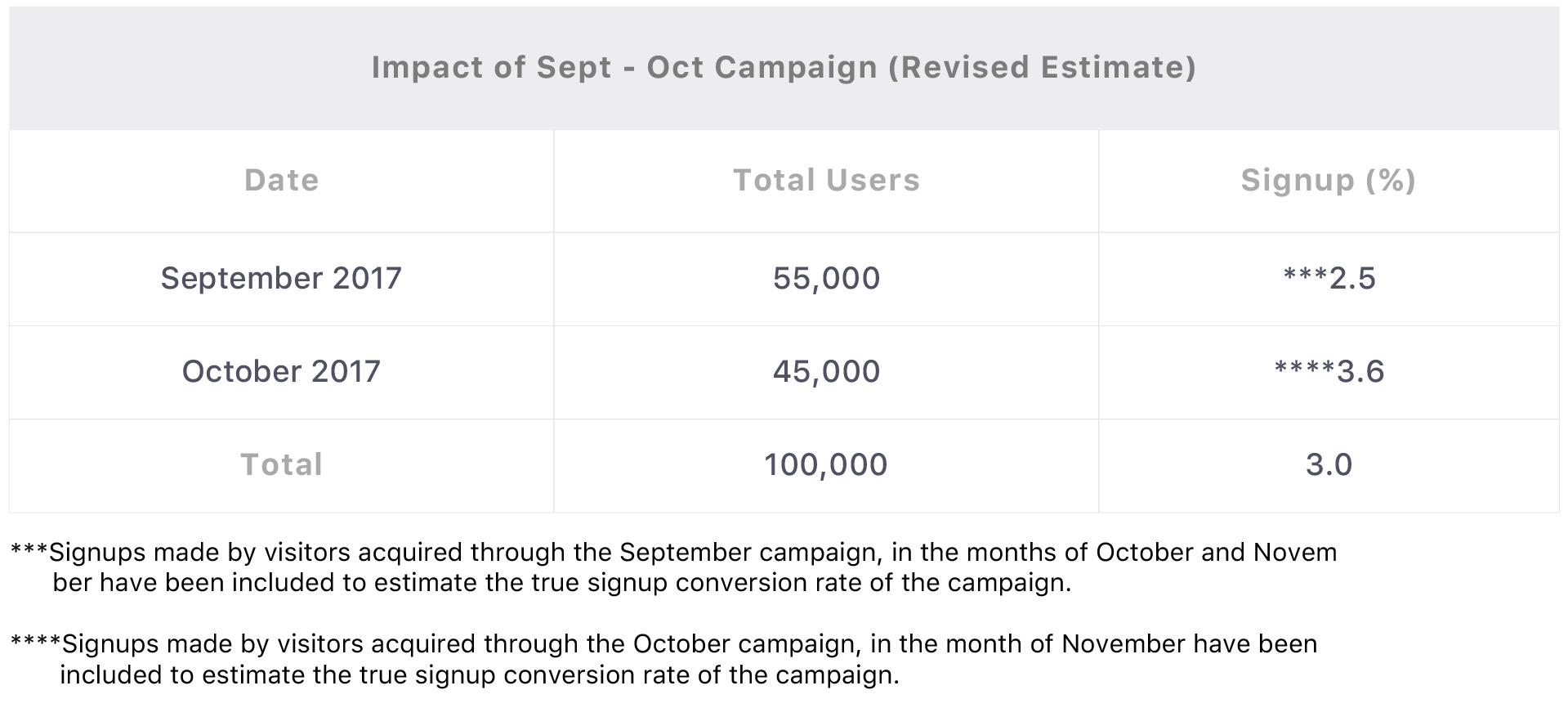
Click to enlarge
Results
This is means that the June ‘17 - July ‘17 campaign led to a 2.5% conversion in signups, while the September ‘17 - October ‘17 campaign resulted in 3% of visitors converting into signups!
If you think about it - you would have never known the true impact of these campaigns had you not done this analysis.
Also, it will be interesting to dig deeper into what you did right in October ‘17 as it has the highest conversion rate, 3.6%!
We hope this has equipped you with a host of ideas to leverage cohorts as a tool to refine your marketing strategy.
Updated almost 4 years ago
Here's a step-by-step guide to analyzing cohorts in your dashboard.